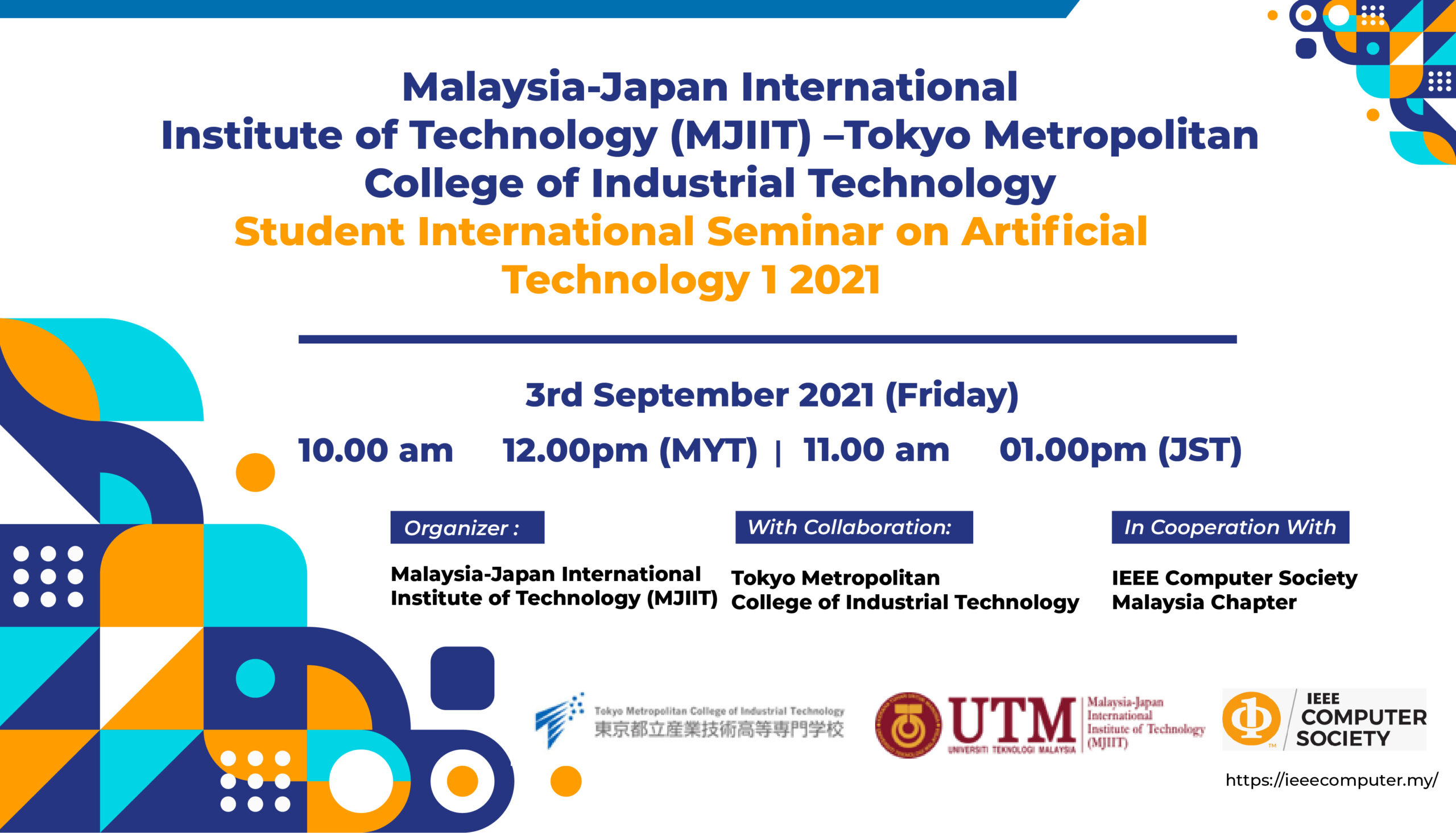
Program Itinerary
Time |
Events |
09.30 – 10.00 |
Registration Registration Form Link: https://bit.ly/RegistrationMJIIT_TMCIT |
10.00 –10.10 |
Welcoming Remark by: Assoc Prof. Dr. Takeru Yokoi |
10:10 – 10:20 |
Project Showcase by TMCIT & MJIIT – Video (Research Video + Corporate Video) *photo session |
10.20 – 11.40 |
Presentation session |
11.50 – 12.00 |
Closing Remarks by: MJIIT Dean: Prof. Ali Selamat Feedback Form Link: https://bit.ly/FeedbackMJIIT_TMCIT |
Presentation Slot
No |
Title Research |
Presenter |
Time (MYT) |
1 |
Phishing Webpage Classification via Deep Learning-based Algorithms: An Empirical Study |
Nguyet Quang Do (MJIIT)
|
10.20 – 10.40 am |
2 |
Creating Highlights with Time-Synchronized Comments in Live Broadcasting |
Natsuki Tsutsui (TMCIT)
|
10.40 – 11.00 am |
3 |
Creating a corpus of claim expressions in tweets |
Takumi Yamagata (TMCIT)
|
11.00 – 11.20 am |
4 |
Machine learning-based attack detection in IoT: Taxonomy, performance evaluation, and future challenges |
Shilan S. Hameed (MJIIT)
|
11.20 – 11.40 am |
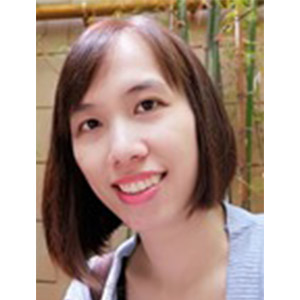
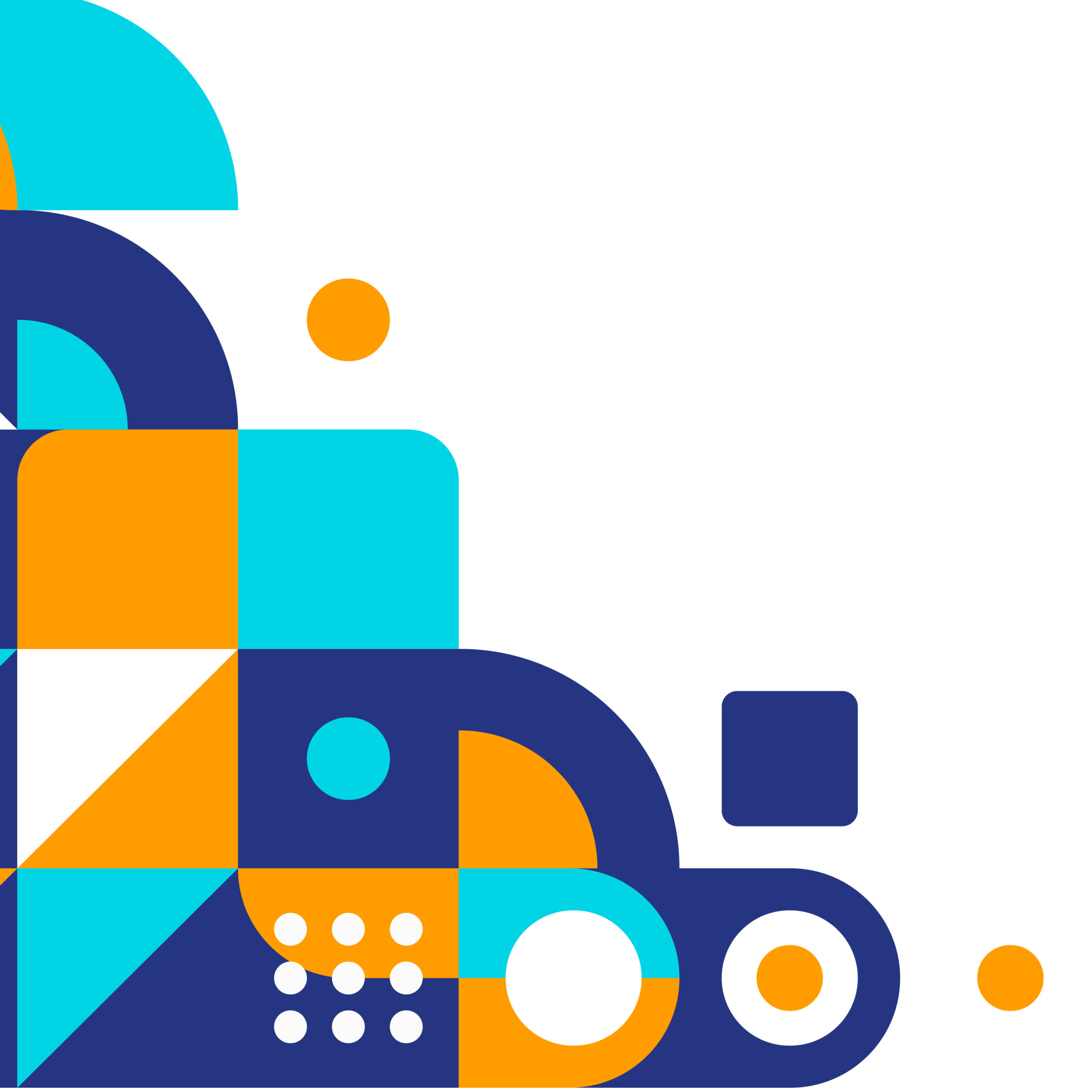
Abstract
Phishing Webpage Classification via Deep Learning-based Algorithms: An Empirical Study
by Nguyet Quang Do
ABSTRACT Phishing detection with high accuracy has always been a topic of great interest. In recent years, new technologies have been developed to improve the phishing detection rate. However, one solution is insufficient to address all problems caused by attackers in cyberspace. Therefore, the primary objective of this paper is to analyze the performance of various deep learning algorithms in detecting phishing activities. Thereby, it will help organizations or individuals select and adopt the proper solution according to their technological needs and specific applications’ requirements to fight against phishing attacks. In this regard, an empirical study was conducted using four different deep learning algorithms, including Deep Neural Network (DNN), Convolutional Neural Network (CNN), Long Short-Term Memory (LSTM), and Gated Recurrent Unit (GRU). To analyze the behaviors of these deep learning architectures, extensive experiments were carried out to examine the impact of parameter settings on the performance accuracy of the deep learning models. The results obtained from the experiments showed that CNN is the best option since it achieved almost equivalent accuracy level as the other three algorithms in the least amount of time. The empirical findings from this paper also manifest several issues and suggest future research directions related to deep learning in the phishing detection domain.
INDEX TERMS Phishing detection; deep learning (DL); Deep Neural Network (DNN); Convolutional Neural Network (CNN); Long Short-Term Memory (LSTM); Gated Recurrent Unit (GRU)
Abstract
Creating Highlights with Time-Synchronized Comments in Live Broadcasting
by Natsuki Tsutsui
ABSTRACT Recently, the number of users of video distribution services such as Youtube has been increasing rapidly. Among them, Twitch, which is limited to live broadcasting and archiving of games, has also been growing significantly. However, it takes a lot of time to watch live broadcasts and archives, and users can only watch a part of the videos because their time is limited. Therefore, we thought that we could increase the number of users and improve their satisfaction by creating video highlights. In this study, we aim to automatically create highlight videos by extracting the excitement of viewers using time-synchronized comments in live broadcasts such as Twitch. Existing research has proposed a method for creating highlights by defining scenes with many comments in a video as the height of the video. However, comments in live broadcasting include irrelevant ones and questions, and it is considered that a scene with many comments is not necessarily a lively scene. Therefore, in this study, we define the event scene in the live broadcast as the excitement, and assume that the comments in the excitement scene are similar. We are planning to create highlights by collecting comments on event scenes from past videos and calculating the similarity of the comments to each scene in the target video.
INDEX TERMS video highlights, live broadcast, Ff-Idf, cosine similarity.
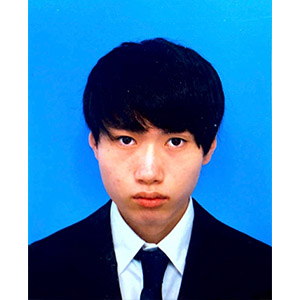
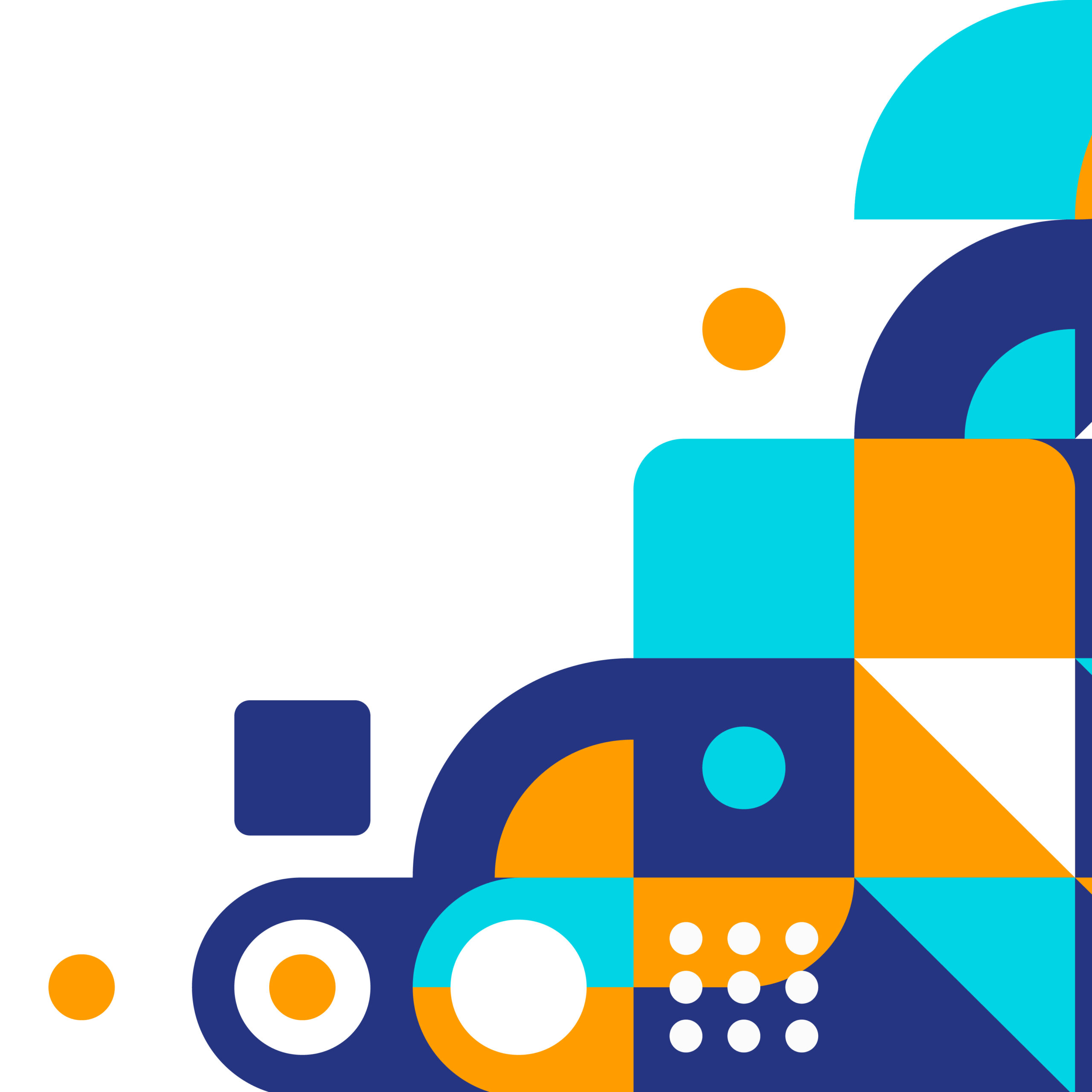
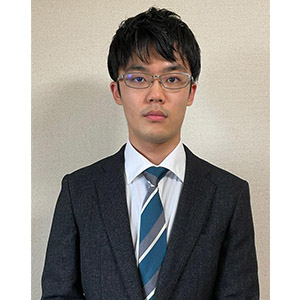
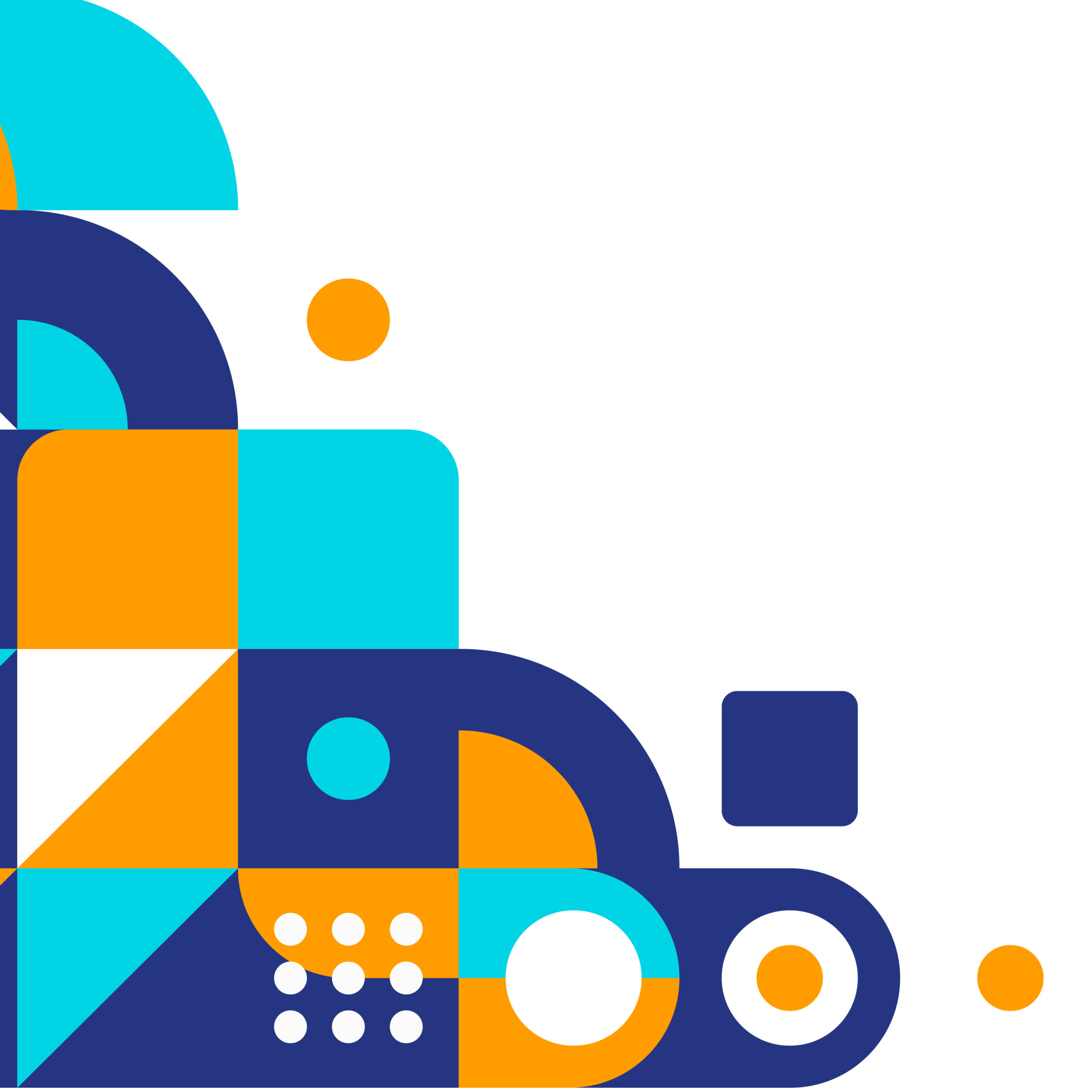
Abstract
Creating A Corpus of Claim Expressions in Tweets
by Takumi Yamagata
ABSTRACT In recent years, the ‘posting’ and ‘spreading’ of false information and fake information on microblogging such as Twitter has become a problem. In particular, the act of spreading information is not only mischievous, but is often done with good intentions. In order to prevent the spread of false information, it is important for the recipient of the information or the person who is trying to spread the information to confirm the authenticity of the information. In order to spot Fake News, which contains false information, journalists use a tool called “fact checking” as a means to combat misinformation. It extracts factors such as claims, claimants, and verdicts (true or false), and shares these factors with other relevant news information to determine the authenticity of the news being investigated. In other words, the extraction of false information requires the investigation of factors such as claims, claimants, and verdicts. However, no method has been proposed to extract Japanese claim expressions for short sentences such as tweets. Therefore, the purpose of this study is to extract claims from tweets based on a rule base, to determine whether they are appropriate as claims, and to create a corpus of claim expressions.
INDEX TERMS Microblogging, Fact check, Claim expressions, Create a corpus..
Abstract
Machine Learning-Based Attack Detection in IoT: Taxonomy, Performance Evaluation, and Future Challenges
by Shilan S. Hameed
ABSTRACT The lack of sufficient security measures and protocol has made the internet of things (IoTs) devices and networks to be at high risk to the emerging cyber-attacks. Machine learning (ML)/deep learning (DL) techniques have been widely employed to solve the issues of attack detection. However, IoT devices and networks cannot handle the resource-extensive ML and DL techniques. Furthermore, the IoT ad hoc, distributed and heterogeneous network characteristics require careful implementation of such detection systems. Therefore, it is important to reveal how these techniques can be effectively utilized for attack detection in IoT, followed by their performance evaluation and limitations. In this paper, we analyze and discuss different aspects of IoT attack detection using ML techniques, including the taxonomy of current IoT cyberattacks and the taxonomy of the current ML techniques for IoT attack/anomaly detection. Hence, state-of-the-art of using ML and DL is elaborated for attack detection in the IoT and evaluating their compatibility to the IoT nature and system in terms of performance metrics, architecture, datasets, and deployment. Furthermore, the main gaps of the studies are highlighted, followed by establishing the future challenges and directions. It was concluded that recent attack detection systems are mainly designed for conventional network systems and hence they have to be appropriately tuned to satisfy IoT systems’ requirements. Additionally, the IoT attack detection approaches encounter several gaps regarding compatibility to the IoT nature and its system. There are some efforts made to incorporate lightweight, real-time, distributed, and scalable detection systems. However, their applicability and performances were not approved. Consequently, we proposed two different attack detection approaches for the IoT networks that can be used for the fog and distributed computing. The online and offline machine learning techniques were compared using the current IoT attack datasets. Results showed that our proposed model outperformed those reported in literature. The legitimacy of experimental results confirmed that a real-time, distributed, and lightweight approach is necessary for the IoT attack detection.
INDEX TERMS Review, Machine Learning, Cyber-Attack, Anomaly, Performance, IoT.
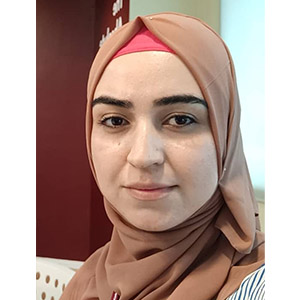
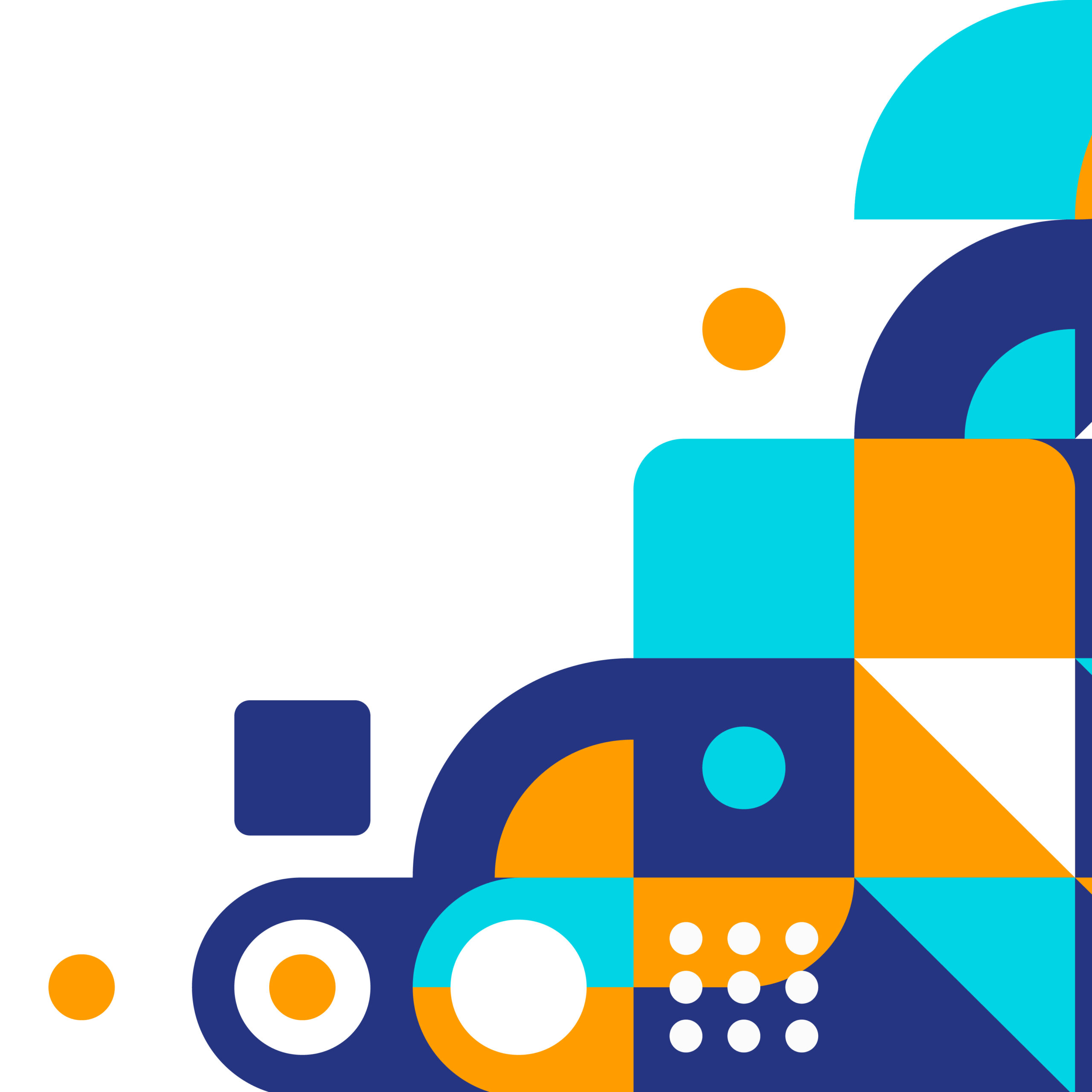