KEYNOTE SPEAKER 1

Hamido Fujita Life Senior Member IEEE, is Executive Chairman of i-SOMET Incorporated Association, Japan, Professor at Malaysia-Japan International Institute of Technology of Universiti Teknologi Malaysia, and Distinguished Professor at Iwate Prefectural University, Japan, he is also Research Professor at University of Granada, Spain. He is Highly Cited Researcher in Cross-Field for the year 2019 and in Computer Science for the year 2020, 2021, 2022, and 2023 respectively, by Clarivate Analytics. He received Doctor Honoris Causa from Óbuda University, Budapest, Hungary, in 2013 and received Doctor Honoris Causa from Timisoara Technical University, Timisoara, Romania, in 2018, and a title of Honorary Professor from Óbuda University, in 2011. He is Distinguished Research Professor at the University of Granada, and Adjunct Professor with Taipei Technical University, Taiwan, Harbin Engineering University, China and others. He supervised Ph.D. students jointly with the University of Laval, Quebec City, QC, Canada; University of Technology Sydney; Oregon State University, Corvallis, OR, USA; University of Paris 1 Pantheon-Sorbonne, Paris, France; and University of Genoa, Italy. Dr. Fujita is the recipient of the Honorary Scholar Award from the University of Technology Sydney, in 2012. He was the Editor-in-Chief for Knowledge-Based Systems (Elsevier) (2005-2019) and then Emeritus Editor of Knowledge-Based Systems in 2020~. Since 2020 he is currently the Editor-in-Chief of Applied Intelligence (Springer) and the Editor-in-Chief of International Journal of Healthcare Management (Taylor & Francis). He headed a number of projects including intelligent HCI, a project related to mental cloning for healthcare systems as an intelligent user interface between human-users and computers, and SCOPE project on virtual doctor systems for medical applications. He collaborated with several research projects in Europe, and recently he is collaborating in OLIMPIA project supported by Tuscany region on Therapeutic monitoring of Parkinson disease. He has published more than 480 articles mainly in high impact factor journals.
On Artificial Intelligence and Machine Learning: Perspectives on Intelligent Health Care Systems
Talk outline: The hot topics in training in Machine Learning is a crucial aspect that affects the credibility of the system in terms of performance and is employed for robust applications such as in healthcare systems. Machines or algorithms, in wide challengeable applications in security or vision or health care early predictions, learn from data. Nevertheless, in most cases, the extensive and unbalanced data and noise make it unreliable in prediction accuracy. Supervised machine learning is and was one of the aspects of providing artificial intelligence-based solutions. However, this is and was limited due to the difficulty of labeling big data and many crucial problems in weak relations and noise in data. Semi-supervised learning, for example, Multiview learning, could assist in solving these problems. In many published research, there are still problems in providing machine learning models that are unbiased and efficient in terms of robustness and resilience in data-driven systems. Multiclass classification still has problems in terms of clear definition in class classification, bias, imbalance and weak relations, making machine learning for multiclass classification insecure for classification or regression analytics. This causes limitations in applying such technology in medical applications and diagnosis prediction. In this lecture, I will outline these problems in our one-class classification project. These are related to providing more robust accuracy prediction with some uncertainty that can help us have more accurate classification and prediction. We have applied such findings in health care for heart sickness and seizure early prediction.
We also have deep learning models, which also have challenges related to evidential deep learning and fairness relative to data. There are important issues in expanding research in evidential deep learning, in which uncertainty prediction of variational Auto encoders can provide decisions on evidential distribution, which in turn helps to provide a measure of uncertainty in decision.
We currently have a research project titled “Healthcare Risk Prediction on Data Streams Employing Signal Transformation Network (OCSTN)”, which is supported by grant from Japan Science Promotion Society (JSPS). In this project, we have employed one-class classification deep neural network for health care prediction. In this lecture I will outline of these perspectives and discuss challenging trends.
KEYNOTE SPEAKER 2
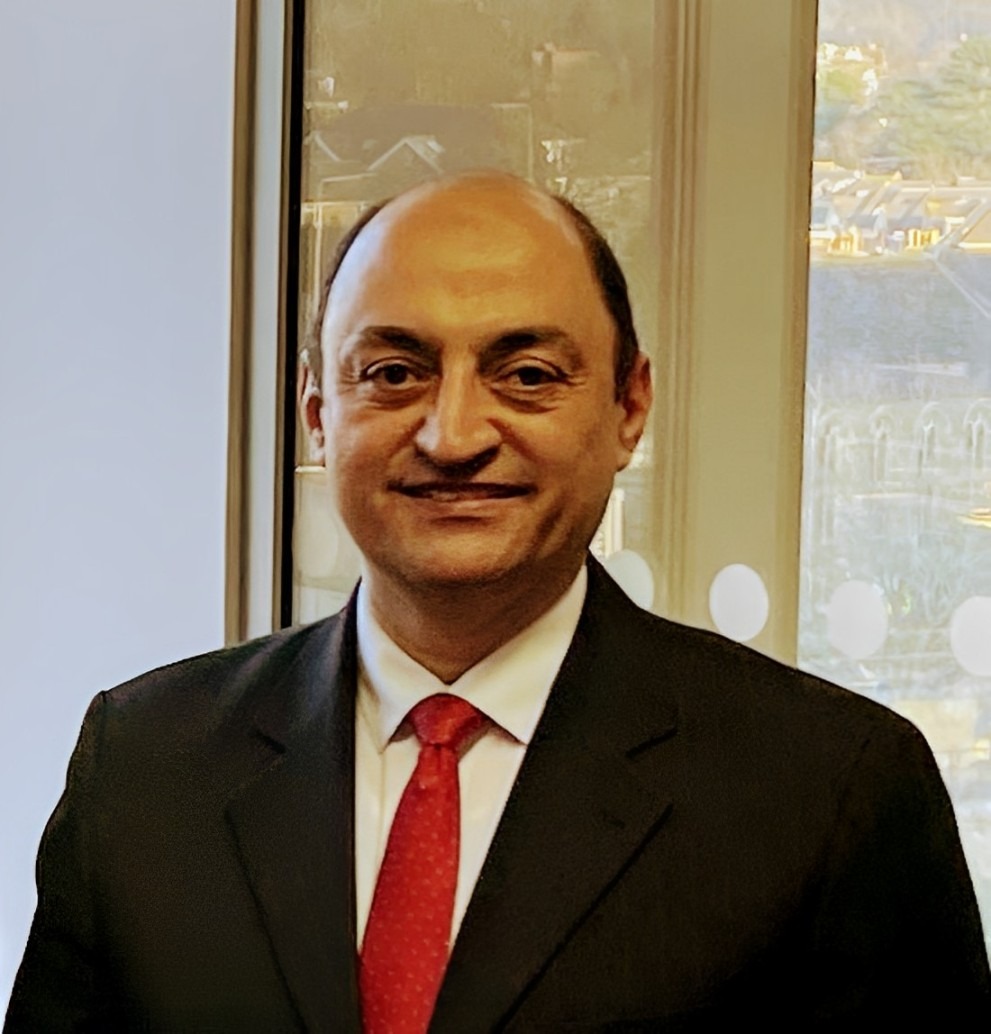
Professor Dr. Yahya Zakaria Mohasseb
The Arab Academy for Science, Technology and Maritime Transport (AASTMT)
Yahya Zakaria Mohasseb, Ph.D., is a Professor of Computer and Communications Engineering at The Arab Academy for Science, Technology and Maritime Transport (AASTMT). Within an illustrious career spanning over three decades, he has made significant contributions to academia, engineering, and educational leadership. Prof. Mohasseb’s research interests encompass the wide area of wireless communications, focusing on channel modeling, network information theory, error control coding, cross-layer optimization, and security. He has supervised over 25 Master and Ph.D. theses and has numerous journal and conference publications. In addition to his academic pursuits, Prof. Mohasseb boasts extensive practical experience in space technology, earth observation, and communications satellites. His expertise extends to the development and implementation of location-aware command and control systems, emphasizing the integration of geospatial technologies into operational frameworks. Prof. Mohasseb earned his bachelor’s and master’s degrees from the Military Technical College (MTC) in Cairo, Egypt, in 1991 and 1996, respectively. He then earned his Ph.D. from The Ohio State University in Columbus, Ohio, USA, in 2002 on a fellowship granted by the Egyptian government . As a full professor for nearly a decade, Prof. Mohasseb has held various leadership positions, contributing to institutional advancement and academic excellence. At MTC, he served as the Dean of Faculty and Deputy Commander. At AASTMT, he assumed the roles of the Dean of Education and Dean of Postgraduate Affairs. Prof. Mohasseb was inducted to the Space and Remote Sensing Research Council of Egypt’s Academy of Scientific Research and Technology. A senior member of the IEEE, he is also the recipient of the Medal of Distinguished Service and The Order of the Republic from the Arab Republic of Egypt.
Artificial Intelligence for 6G: PHY Layer or Networked Intelligence?
6G marks a significant leap forward from its predecessors, offering dramatic improvements in speed, latency, and capacity. These advancements pave the way for transformative applications across diverse sectors. Central to unlocking 6G’s potential is the integration of Artificial Intelligence (AI) throughout its architecture. AI acts as a driving force, enhancing performance across all layers and functions of the network. From network orchestration and management to complex signal processing at the Physical Layer, AI algorithms optimize resource allocation, personalize user experiences, and tailor signaling and channel access techniques. For the physical layer, AI applications go beyond traditional tasks like channel estimation, pioneering techniques like passive beamforming and index modulation. AI also has the capability to process data at the network edge, facilitating real-time decision-making in critical, time-sensitive applications. This capability is indispensable to enabl explosion of connected devices, empowering smart devices and robots, and driving groundbreaking applications in healthcare, manufacturing, transportation, and agriculture. In this talk, we will explore the transformative potential of AI in the realm of 6G. We’ll also evaluate AI solutions based on key metrics like efficiency, interpretability, reliability, and cost- effectiveness. To guide our discussion, we will consider these fundamental questions:
• How do interpretability and reliability of AI solutions impact their adoption in critical applications for 6G?
• Are there areas within 6G where the use of AI should be discouraged or even excluded?
• How can industry stakeholders collaborate to ensure the responsible development and deployment of AI-enabled 6G technologies?
• Will the use of AI help or hinder standardization efforts for 6G and beyond?
KEYNOTE SPEAKER 3

Dr. Ir. M Zurix Mohamed
Petroliam Nasional Berhad (PETRONAS)
Dr. Ir. M Zurix Mohamed is the distinguished Custodian for Instrument & Control, specializing in Measurement within PETRONAS Group Technical Solutions (GTS). With a profound 20 years of industry experience, he has made significant strides in refinery operations, mega projects, and the advancement of digital tool development, positioning him as a pivotal figure in PETRONAS’s journey towards innovation and operational excellence. Holding the prestigious title of Professional Engineer recognized by the Board of Engineers Malaysia, Dr. Zurix has augmented his professional standing with a PhD in Engineering Management. Since joining PETRONAS in 2003 as a Maintenance Engineer, he has ascended through the ranks, demonstrating exceptional leadership and technical acumen.
Dr. Zurix’s tenure is marked by his leadership in the development of multiple PETRONAS technologies, all of which are IP-patented and now integral to the functionality of oil and gas plants. His commitment to innovation extends to plant optimization, leveraging digital tools and artificial intelligence to enhance operational efficiency. His efforts have not only led to the patenting of various optimization technologies in instrument and control but have also positioned PETRONAS at the forefront of technological advancements in the industry. As an active contributor to the engineering community, Dr. Zurix regularly engages in multiple conferences, sharing his insights and breakthroughs. His exceptional work has been recognized with multiple awards, celebrating his contributions to instrument and control engineering and his impactful role in developing cutting-edge technologies for PETRONAS.